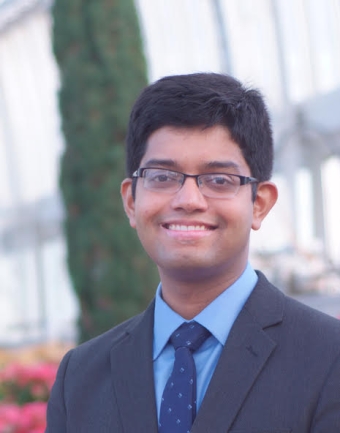
Date:
Location:
Title:
Abstract: The ground state electronic structure of a material — obtainable using Kohn-Sham Density Functional Theory (KS-DFT) simulations — contains a vast wealth of information, making it a key unknown in computational materials science. It can be used to compute structural parameters, elastic constants, the electronic density of states, magnetic properties, etc., and can also serve as the starting point for calculations of excited state phenomena, including those related to optical and transport properties.
In this talk, I will describe our work on combining specialized first principles calculations with state-of-the-art machine learning (ML) techniques, to enable electronic structure prediction of various nanomaterials and bulk systems, while making such predictions systematic, reliable and efficient. I will focus on two related but independent directions. First, I will present how symmetry-adapted density functional theory calculations can be used to train interpretable ML models for the electronic fields of chiral nanomaterials. Using distorted carbon nanotubes as a case study, I will demonstrate how this framework achieves high accuracy even with limited training data, and how it can be leveraged for automated materials discovery. Second, I will discuss how high-throughput KS-DFT calculations can be used to train ML models for chemically disordered bulk systems. I will illustrate their application in predicting the electronic structure across the composition space of medium- and high-entropy alloys.
I will conclude by demonstrating how our framework pushes the frontier of electronic structure calculations by enabling multi-million atom calculations routinely with only modest computational resources. At the same time, it reveals hitherto unidentified features of the electronic properties of materials, including startling degrees of redundancy in electronic structure data. I will close with a brief overview of ongoing and future directions.
Hosted by Ananya Renuka-Balakrishna.